Contents
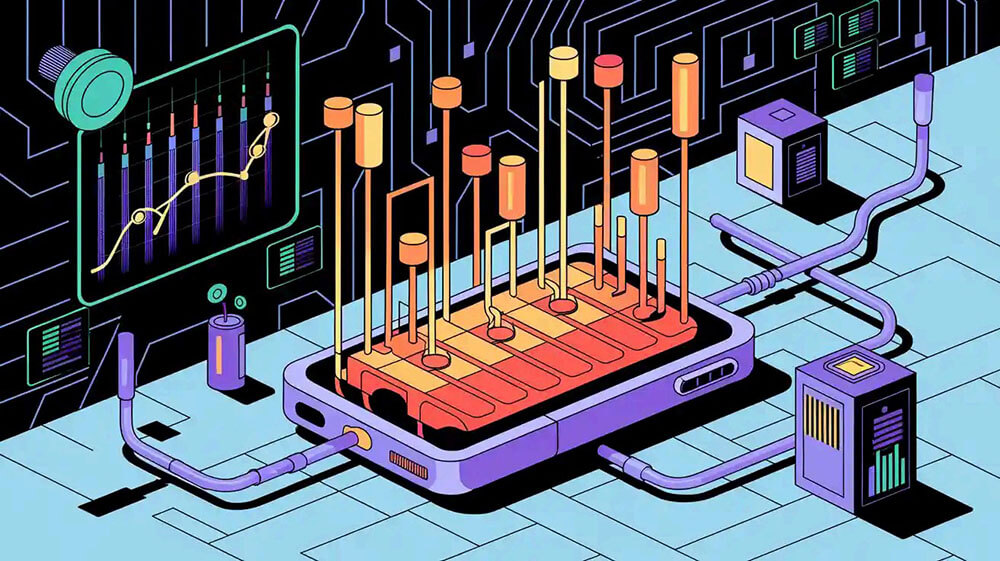
Data-driven models and lithium-ion battery technology serve as powerful tools for analyzing and forecasting battery performance. By leveraging algorithms like the Improved Random Forest (IRF), these models achieve unmatched accuracy, with an RMSE of 1.58 and an R² score of 0.9995. Such precision transforms battery health monitoring, mitigating battery capacity fade and extending battery longevity. These advancements are crucial for managing degraded lithium-ion batteries and forecasting battery lifetime in applications like robotic and renewable energy systems.
Key Takeaways
Data-driven models help lithium-ion batteries work better by using smart math to guess how they will act.
Correct State-of-Charge (SOC) guesses are important for battery safety and working well, helping areas like robots and gadgets.
Mixing data-driven and model-based methods makes batteries last longer and work better, aiding green energy efforts.
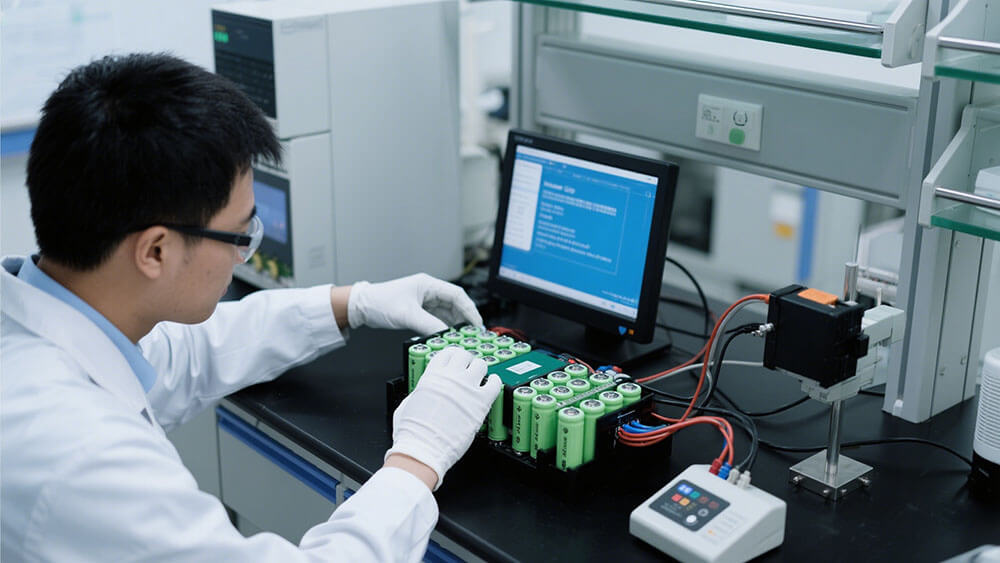
Part 1: Core Components of Data-Driven Models and Lithium-Ion Battery Technology
1.1 Data Collection and Preprocessing for Lithium-Ion Batteries
Data collection and preprocessing form the foundation of any data-driven model for lithium-ion batteries. You must ensure that the data collected is accurate, consistent, and representative of real-world conditions. Key factors influencing the quality of lithium-ion battery cells include electrode uniformity, component dryness, and precise alignment of electrodes. For instance, maintaining consistent electrolyte amounts across batches is critical for reproducibility. Excessive electrolyte variations during assembly can lead to inconsistent results, impacting the model’s reliability.
Pre-drying components and rinsing them with acetone or DI water helps remove residues, ensuring cleaner data inputs. Proper alignment of the cathode and anode is equally important. Misalignment can compromise cycling stability, which directly affects the accuracy of lifetime prediction models. Internal pressure, controlled through spacer thickness, also plays a vital role in achieving reproducible results. By addressing these factors, you can enhance the quality of data used in your models, leading to more reliable predictions.
1.2 Machine Learning Algorithms in Data-Driven Models
Machine learning algorithms are at the heart of data-driven models for lithium-ion batteries. These algorithms analyze vast datasets to uncover patterns and relationships that traditional methods might overlook. For example, AI-based models have achieved a mean absolute error (MAE) of less than 1.27 milliampere-hours (mAh) and a root mean square error (RMSE) under 1.43 mAh. Such precision demonstrates the robustness of these models across various environmental conditions.
Support Vector Regression (SVR), Relevance Vector Machine (RVM), and Gaussian Process Regression (GPR) are commonly used techniques. These methods excel in learning from historical and real-time data, enabling accurate predictions of battery performance. Hybrid approaches, such as combining SVR with Long Short-Term Memory (LSTM) models, further enhance prediction accuracy. Comprehensive evaluations confirm their effectiveness in predicting capacity and remaining useful life (RUL). By leveraging these algorithms, you can optimize battery performance and extend its operational lifespan.
1.3 Predictive Methodologies for Battery Behavior Analysis
Predictive methodologies play a crucial role in understanding and forecasting the behavior of lithium-ion batteries. Model-based methods, including electrochemical and equivalent circuit models, simulate battery behavior to provide insights into degradation mechanisms. These methods are validated through numerical simulations and statistical error analyses, ensuring their reliability.
Data-driven methodologies complement model-based approaches by using machine learning techniques to analyze historical and real-time data. For instance, hybrid models that integrate SVR and LSTM have proven effective in accurately predicting capacity and RUL. Performance indicators such as Final Area-Specific Impedance (ASI) and Final Specific Capacity (Q) further validate these methodologies. Lower ASI values indicate higher power density and efficiency, while higher Q values represent greater energy storage capacity.
By combining model-based and data-driven approaches, you can achieve a comprehensive understanding of battery behavior. This integration not only enhances prediction accuracy but also supports the development of more efficient and durable lithium-ion batteries.
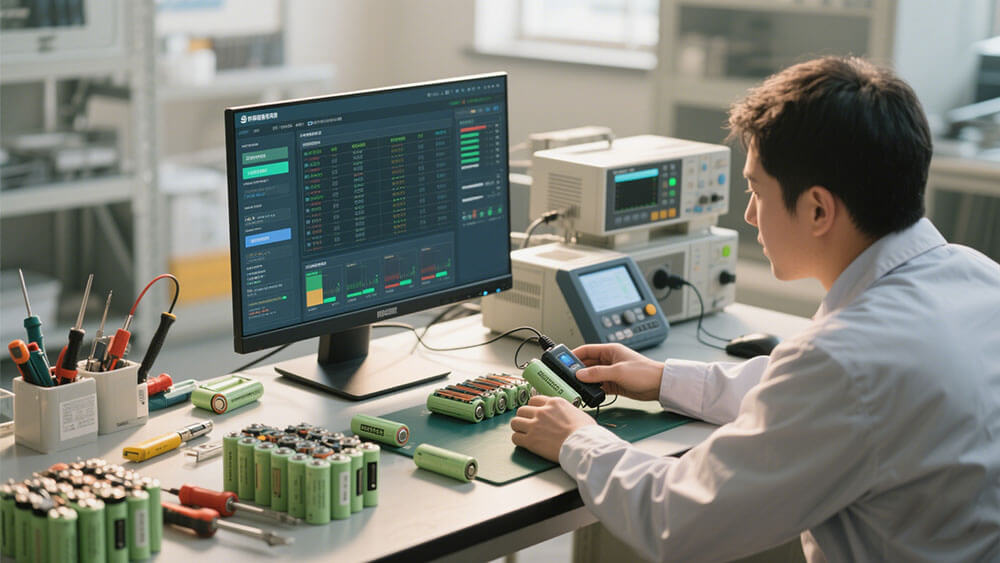
Part 2: Key Applications of Data-Driven Models in Lithium-Ion Batteries
2.1 State-of-Charge (SOC) Prediction for Battery Packs
Accurate State-of-Charge (SOC) prediction is essential for ensuring the operational safety and efficiency of lithium-ion battery packs. Data-driven models excel in this area by leveraging advanced algorithms to analyze real-time and historical data. These models provide precise SOC estimates, which are critical for applications in industries like robotics, infrastructure, and consumer electronics. For example, in robotics, reliable SOC predictions ensure uninterrupted operations, enhancing productivity and reducing downtime.
Data-driven models achieve remarkable predictive performance in SOC estimation.
Predictors have demonstrated RMSE values as low as 5×10⁻⁷ and 1.3×10⁻³ for simulated data.
On experimental battery measurements, RMSE values of 2.2×10⁻⁶ and 4.8×10⁻⁴ were achieved.
Generalization scores on unseen real-world measurements reached 8.5×10⁻⁴.
These results highlight the models’ ability to adapt to diverse operating conditions. By optimizing hyperparameters, you can further enhance accuracy while minimizing computational complexity. This systematic approach ensures that SOC predictions remain reliable across various environments, from medical devices to industrial machinery.
2.2 Capacity Fade and Degradation Analysis in Lithium-Ion Batteries
Capacity fade analysis is a cornerstone of battery health monitoring. Data-driven models enable you to identify and quantify degradation mechanisms, ensuring optimal performance and extended battery lifespan. This capability is particularly valuable in sectors like medical devices, where consistent power delivery is critical.
Key metrics for capacity fade analysis include:
Metric | Description |
---|---|
Capacity Fade | Remaining usable capacity measured over time under various operating conditions. |
Impedance Measurements | Impedance data collected during check-ups to assess battery health and degradation. |
Data Points | Over 3 billion data points from 228 commercial NMC/C+SiO lithium-ion cells. |
Aging Duration | Cells aged for more than a year to analyze long-term performance. |
Operating Conditions | Data collected under a wide range of driving cycles to understand different aging effects. |
By analyzing these metrics, you can gain insights into degradation patterns and their impact on battery performance. For instance, data-driven models can differentiate between capacity loss due to lithium inventory depletion and active material degradation. This level of detail supports the development of more robust batteries for applications in security systems and industrial equipment.
2.3 Lifetime Prediction for Lithium-Ion Battery Packs
Predicting the lifetime of lithium-ion battery packs is crucial for reducing costs and improving sustainability. Data-driven models offer a probabilistic approach to lifetime prediction, enabling you to forecast capacity degradation and identify the weakest cells in a pack. This capability is particularly beneficial for infrastructure projects, where battery reliability directly impacts operational safety.
Key contributions of data-driven lifetime prediction models include:
Advantage/Contribution | Description |
---|---|
Knowledge Transfer | Transfers knowledge from individual cells to predict pack lifetime without requiring identical degradation patterns. |
Probabilistic Prediction | Provides probabilistic forecasts of capacity degradation using extended health indicators (HIs). |
Capacity Prediction | Identifies inconsistencies and capacity distribution during aging, pinpointing the weakest cell for maintenance. |
Time Efficiency | Reduces aging experiment time by over 85%, accelerating battery development cycles. |
These models not only enhance prediction accuracy but also support proactive maintenance strategies. By identifying potential failures early, you can minimize downtime and extend the operational lifespan of your battery packs.
2.4 Second-Life Battery Classification and Repurposing
As the demand for sustainable energy solutions grows, second-life battery applications have become increasingly important. Data-driven models play a pivotal role in classifying and repurposing used batteries, ensuring their efficient reuse in less demanding applications. For example, retired batteries from electric vehicles can be repurposed for energy storage in industrial or consumer electronics systems.
These models analyze key parameters such as remaining capacity, internal resistance, and cycle history to determine a battery’s suitability for second-life use. By leveraging this data, you can maximize the value of used batteries while minimizing environmental impact. This approach aligns with global sustainability goals and supports the transition to a circular economy.
Data-driven models revolutionize lithium-ion battery technology by delivering precise insights into performance and degradation. These tools empower you to enhance battery efficiency, extend operational life, and contribute to sustainable energy practices. Unlock the full potential of your applications by exploring custom battery solutions tailored to your unique needs.
FAQ
1. What industries benefit most from data-driven models for lithium-ion batteries?
Data-driven models enhance battery performance in industries like medical devices, robotics, security systems, infrastructure, consumer electronics, and industrial applications. These models optimize efficiency and reliability. Explore custom solutions here.
2. How do data-driven models improve battery management systems (BMS)?
These models analyze real-time data to optimize charging, monitor health, and predict failures. This ensures enhanced accuracy, extended lifespan, and reduced downtime for lithium-ion battery packs.
3. Why choose Large Power for custom battery solutions?
Large Power specializes in tailored lithium-ion battery solutions. Our expertise ensures optimal performance, sustainability, and reliability for your specific industry needs.